Why Your AI Team Needs an AI PM (Quality) Lead
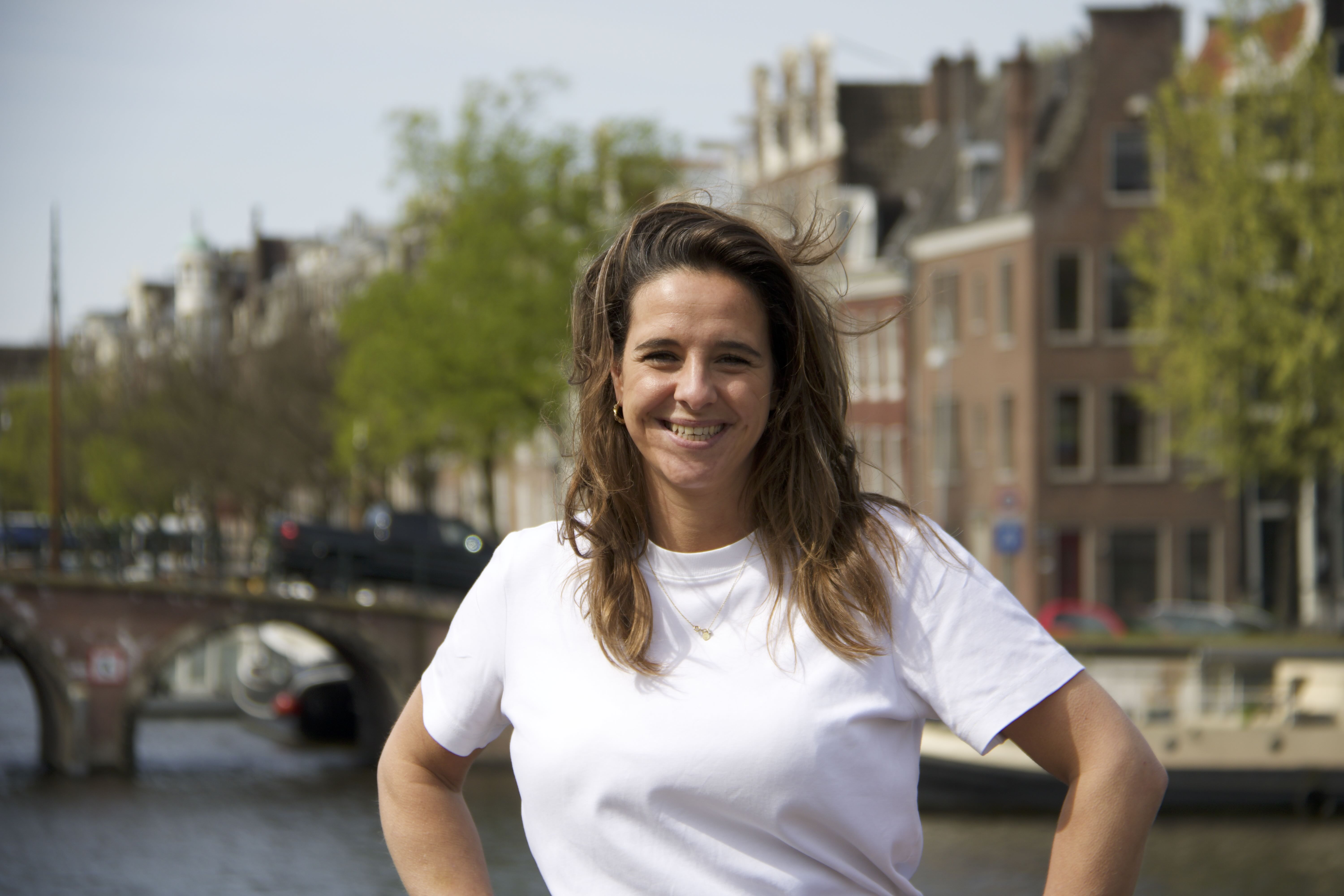
Manouk
Apr 2, 2025
As LLM-driven products mature, it’s no longer enough to rely on traditional PM roles or generic ML oversight. The best GenAI teams are now introducing a critical new role: the AI PM (Quality) Lead.
This isn't your average product manager. This person bridges product, engineering, and annotation workflows — ensuring your LLM product isn’t just functional, but trusted, observable, and continuously improving.
Why “AI Quality” Isn’t Just QA with a fancy name
In traditional software, QA comes in late to validate what’s already built. But in GenAI products, quality has to be baked into the lifecycle — from prompt iteration and system message design to grounding and final outputs.
That’s where the AI PM (Quality) Lead comes in. They’re not just checking boxes — they’re working alongside domain experts to define quality thresholds, design annotation strategies, and prioritize the most impactful feedback loops. Without this role, quality efforts end up scattered across data scientists, PMs, and engineers — and that usually means they’re done inconsistently or not at all.
The hidden cost of ignoring quality
In early-stage GenAI teams, quality is often the thing everyone knows matters, but no one owns. This leads to:
Inconsistent data annotations across evaluators
Endless debates over what “good” looks like
Unclear feedback loops from users to retraining datasets
No way to track regressions or version performance over time
This chaos becomes especially risky in regulated or high-stakes domains, where LLM observability and traceability aren’t just “nice to have” — they’re mandatory.
The AI PM (Quality) role in action
So what does this person actually do?
Define: Work with stakeholders and domain experts to articulate what quality means per use case (factuality? tone? traceability?).
Operationalize: Design annotation flows and guidelines, decide which metrics matter, and integrate tools like LangWatch’s feedback loop into the dev cycle.
Observe: Drive an observability-first culture where product and infra teams can trace outputs back to the inputs, prompts, and model versions that produced them.
Done right, this role unlocks faster iteration, more defensible AI products, and less time wasted debugging “ghost bugs” in your pipeline.
Who should step into this role?
Some teams promote a senior PM, Customer/User Experience Manager or Data Lead into this role. Others bring in someone from an ML research background with a knack for product thinking. What matters most is that they’re obsessed with clarity, annotation workflows, and marrying user feedback with model behavior.
What you can do today
If your team is building with LLMs, ask yourself: who owns quality? If you don’t have a clear answer, it might be time to carve out this role. The earlier you do it, the faster your team will move — with fewer painful rewrites, compliance risks, or customer churn.
Want to go deeper on building feedback loops that actually improve model performance? Check out our docs on Annotations for Human in the loop collaboration.
Or, for inspiration, look at how companies like PHWL.ai have scaled LLM quality as a first-class product function.
Get started for free
As LLM-driven products mature, it’s no longer enough to rely on traditional PM roles or generic ML oversight. The best GenAI teams are now introducing a critical new role: the AI PM (Quality) Lead.
This isn't your average product manager. This person bridges product, engineering, and annotation workflows — ensuring your LLM product isn’t just functional, but trusted, observable, and continuously improving.
Why “AI Quality” Isn’t Just QA with a fancy name
In traditional software, QA comes in late to validate what’s already built. But in GenAI products, quality has to be baked into the lifecycle — from prompt iteration and system message design to grounding and final outputs.
That’s where the AI PM (Quality) Lead comes in. They’re not just checking boxes — they’re working alongside domain experts to define quality thresholds, design annotation strategies, and prioritize the most impactful feedback loops. Without this role, quality efforts end up scattered across data scientists, PMs, and engineers — and that usually means they’re done inconsistently or not at all.
The hidden cost of ignoring quality
In early-stage GenAI teams, quality is often the thing everyone knows matters, but no one owns. This leads to:
Inconsistent data annotations across evaluators
Endless debates over what “good” looks like
Unclear feedback loops from users to retraining datasets
No way to track regressions or version performance over time
This chaos becomes especially risky in regulated or high-stakes domains, where LLM observability and traceability aren’t just “nice to have” — they’re mandatory.
The AI PM (Quality) role in action
So what does this person actually do?
Define: Work with stakeholders and domain experts to articulate what quality means per use case (factuality? tone? traceability?).
Operationalize: Design annotation flows and guidelines, decide which metrics matter, and integrate tools like LangWatch’s feedback loop into the dev cycle.
Observe: Drive an observability-first culture where product and infra teams can trace outputs back to the inputs, prompts, and model versions that produced them.
Done right, this role unlocks faster iteration, more defensible AI products, and less time wasted debugging “ghost bugs” in your pipeline.
Who should step into this role?
Some teams promote a senior PM, Customer/User Experience Manager or Data Lead into this role. Others bring in someone from an ML research background with a knack for product thinking. What matters most is that they’re obsessed with clarity, annotation workflows, and marrying user feedback with model behavior.
What you can do today
If your team is building with LLMs, ask yourself: who owns quality? If you don’t have a clear answer, it might be time to carve out this role. The earlier you do it, the faster your team will move — with fewer painful rewrites, compliance risks, or customer churn.
Want to go deeper on building feedback loops that actually improve model performance? Check out our docs on Annotations for Human in the loop collaboration.
Or, for inspiration, look at how companies like PHWL.ai have scaled LLM quality as a first-class product function.
Get started for free
As LLM-driven products mature, it’s no longer enough to rely on traditional PM roles or generic ML oversight. The best GenAI teams are now introducing a critical new role: the AI PM (Quality) Lead.
This isn't your average product manager. This person bridges product, engineering, and annotation workflows — ensuring your LLM product isn’t just functional, but trusted, observable, and continuously improving.
Why “AI Quality” Isn’t Just QA with a fancy name
In traditional software, QA comes in late to validate what’s already built. But in GenAI products, quality has to be baked into the lifecycle — from prompt iteration and system message design to grounding and final outputs.
That’s where the AI PM (Quality) Lead comes in. They’re not just checking boxes — they’re working alongside domain experts to define quality thresholds, design annotation strategies, and prioritize the most impactful feedback loops. Without this role, quality efforts end up scattered across data scientists, PMs, and engineers — and that usually means they’re done inconsistently or not at all.
The hidden cost of ignoring quality
In early-stage GenAI teams, quality is often the thing everyone knows matters, but no one owns. This leads to:
Inconsistent data annotations across evaluators
Endless debates over what “good” looks like
Unclear feedback loops from users to retraining datasets
No way to track regressions or version performance over time
This chaos becomes especially risky in regulated or high-stakes domains, where LLM observability and traceability aren’t just “nice to have” — they’re mandatory.
The AI PM (Quality) role in action
So what does this person actually do?
Define: Work with stakeholders and domain experts to articulate what quality means per use case (factuality? tone? traceability?).
Operationalize: Design annotation flows and guidelines, decide which metrics matter, and integrate tools like LangWatch’s feedback loop into the dev cycle.
Observe: Drive an observability-first culture where product and infra teams can trace outputs back to the inputs, prompts, and model versions that produced them.
Done right, this role unlocks faster iteration, more defensible AI products, and less time wasted debugging “ghost bugs” in your pipeline.
Who should step into this role?
Some teams promote a senior PM, Customer/User Experience Manager or Data Lead into this role. Others bring in someone from an ML research background with a knack for product thinking. What matters most is that they’re obsessed with clarity, annotation workflows, and marrying user feedback with model behavior.
What you can do today
If your team is building with LLMs, ask yourself: who owns quality? If you don’t have a clear answer, it might be time to carve out this role. The earlier you do it, the faster your team will move — with fewer painful rewrites, compliance risks, or customer churn.
Want to go deeper on building feedback loops that actually improve model performance? Check out our docs on Annotations for Human in the loop collaboration.
Or, for inspiration, look at how companies like PHWL.ai have scaled LLM quality as a first-class product function.
Get started for free
Boost your LLM's performance today
Get up and running with LangWatch in as little as 10 minutes.
Benefits
Features
Boost your LLM's performance today
Get up and running with LangWatch in as little as 10 minutes.
Benefits
Features
Boost your LLM's performance today
Get up and running with LangWatch in as little as 10 minutes.
Benefits
Features