Data Flywheel: Using your production data to build better LLM products

Rogerio - CTO
Jun 27, 2024
It does not come as a shock for anybody when I say that data is one of the most valuable assets a company can have, this has been true for some years now. Valuable data can be used to build very powerful products, and with the invention of LLMs, it became even more obvious that companies that already have valuable data internally can leverage AI to create a bigger edge.
But internal data is not the one I want to talk about today, I want to talk about the data that is produced as a byproduct of your AI being in production: all the interactions your users have with it, both with happy and unhappy outcomes. This is super valuable data, it’s literally the best insight on how users expect your product to behave, and now it comes in conversational text format, it's not just numbers like traditional product analytics, so it can directly feed back into the AI.
The thing with LLMs, is that they are super powerful and general, this is the exact reason we want to use them that, they can answer any questions and execute a large range of tasks, however exactly because of that, their surface area to the real world usage is extremely large, the more they do the larger it is, resulting in countless different ways it can fall short. It is hopeless to try and predict all the ways your users will want to use your product, and try covering all the possibilities and edge cases beforehand.
How can a Data Flywheel help LLM apps?
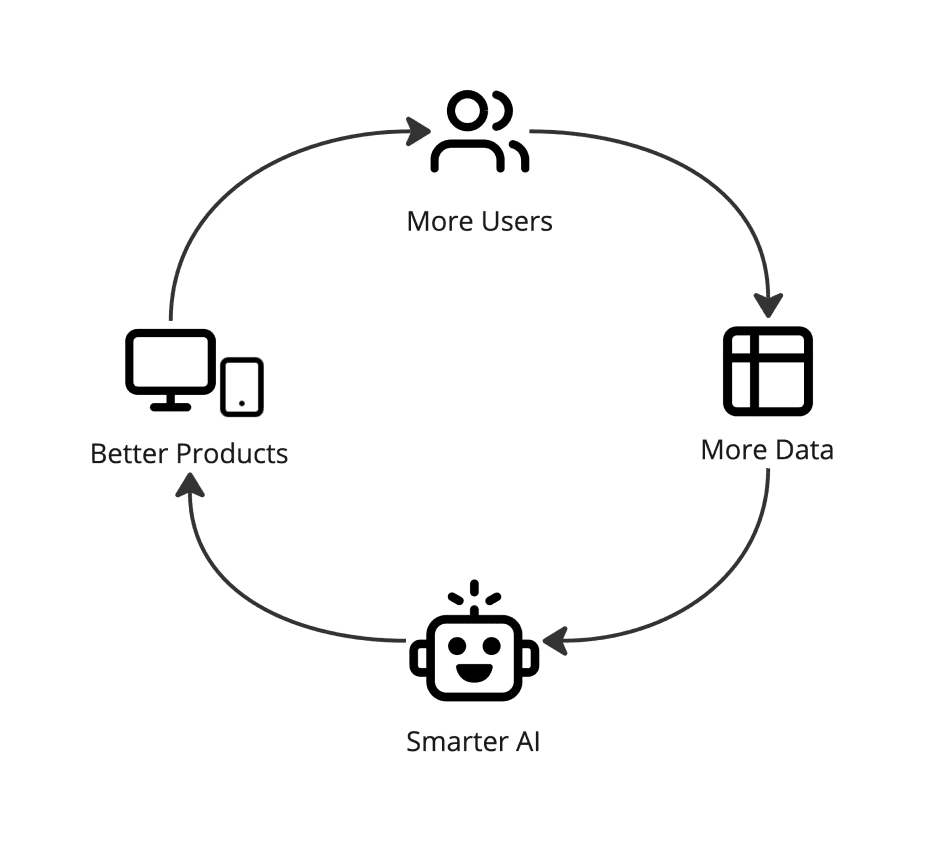
We call it a flywheel when an improvement brings business value and that loops back on further improvements. Since the number of possibilities of an LLM product is too vast - think of all possible ways a user can try using your product, or even compose a sentence - nothing is better at selecting what is more valuable and relevant like the real world, this makes it essential to monitor and get insights on where the product is suboptimal, or where it could bring even more value.
Then by using those insights for improving the product and making it more valuable, it will receive more usage and more users, it will gain more trust and cover more cases to be used in more places. By getting more usage, even more scenarios are revealed, new paths to bring even more value, and new shortcomings to overcome and make the product more robust, which will lead to even more usage an so on. That’s the flywheel.
The advantage with AI over traditional software improvements, is that this connection is even more direct, LLMs can be used also to evaluate, classify and synthesize data that will improve the product, so this flywheel is closed even tighter together.
There is, however, no shortcuts, if your solution has been finding new unexpected edge cases for months or years, it is not possible for someone else to just guess which ones they are and port them over elsewhere without going through the same discovery process. The improvement process you done on your product for your specific domain then creates an edge that sets you apart from others as soon as you start it.
LangWatch
LangWatch was built on top of this realization, to help you maximize your data flywheel. Our platform helps both on collection part, with monitoring, evaluations, insights, collecting user feedback and annotating with domain experts from your team, and on the improvement part, running experiments, validating and guaranteeing the improved quality of each iteration.
Interested how it can help your GenAI solution? Book a demo with us.
It does not come as a shock for anybody when I say that data is one of the most valuable assets a company can have, this has been true for some years now. Valuable data can be used to build very powerful products, and with the invention of LLMs, it became even more obvious that companies that already have valuable data internally can leverage AI to create a bigger edge.
But internal data is not the one I want to talk about today, I want to talk about the data that is produced as a byproduct of your AI being in production: all the interactions your users have with it, both with happy and unhappy outcomes. This is super valuable data, it’s literally the best insight on how users expect your product to behave, and now it comes in conversational text format, it's not just numbers like traditional product analytics, so it can directly feed back into the AI.
The thing with LLMs, is that they are super powerful and general, this is the exact reason we want to use them that, they can answer any questions and execute a large range of tasks, however exactly because of that, their surface area to the real world usage is extremely large, the more they do the larger it is, resulting in countless different ways it can fall short. It is hopeless to try and predict all the ways your users will want to use your product, and try covering all the possibilities and edge cases beforehand.
How can a Data Flywheel help LLM apps?
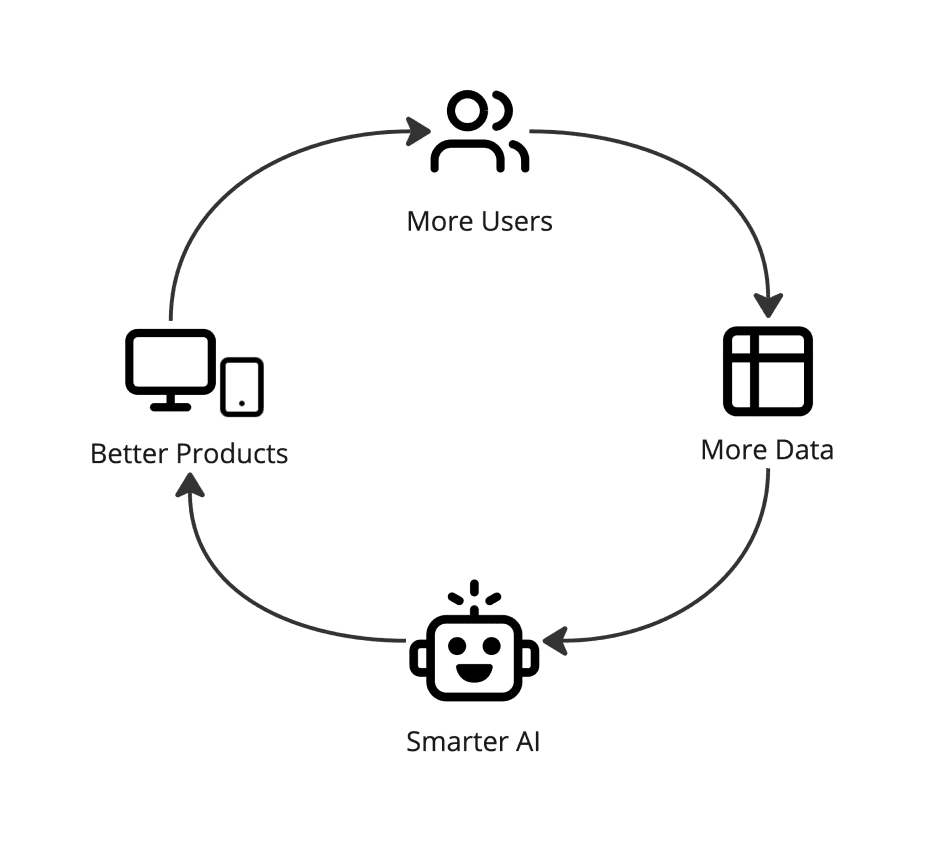
We call it a flywheel when an improvement brings business value and that loops back on further improvements. Since the number of possibilities of an LLM product is too vast - think of all possible ways a user can try using your product, or even compose a sentence - nothing is better at selecting what is more valuable and relevant like the real world, this makes it essential to monitor and get insights on where the product is suboptimal, or where it could bring even more value.
Then by using those insights for improving the product and making it more valuable, it will receive more usage and more users, it will gain more trust and cover more cases to be used in more places. By getting more usage, even more scenarios are revealed, new paths to bring even more value, and new shortcomings to overcome and make the product more robust, which will lead to even more usage an so on. That’s the flywheel.
The advantage with AI over traditional software improvements, is that this connection is even more direct, LLMs can be used also to evaluate, classify and synthesize data that will improve the product, so this flywheel is closed even tighter together.
There is, however, no shortcuts, if your solution has been finding new unexpected edge cases for months or years, it is not possible for someone else to just guess which ones they are and port them over elsewhere without going through the same discovery process. The improvement process you done on your product for your specific domain then creates an edge that sets you apart from others as soon as you start it.
LangWatch
LangWatch was built on top of this realization, to help you maximize your data flywheel. Our platform helps both on collection part, with monitoring, evaluations, insights, collecting user feedback and annotating with domain experts from your team, and on the improvement part, running experiments, validating and guaranteeing the improved quality of each iteration.
Interested how it can help your GenAI solution? Book a demo with us.
It does not come as a shock for anybody when I say that data is one of the most valuable assets a company can have, this has been true for some years now. Valuable data can be used to build very powerful products, and with the invention of LLMs, it became even more obvious that companies that already have valuable data internally can leverage AI to create a bigger edge.
But internal data is not the one I want to talk about today, I want to talk about the data that is produced as a byproduct of your AI being in production: all the interactions your users have with it, both with happy and unhappy outcomes. This is super valuable data, it’s literally the best insight on how users expect your product to behave, and now it comes in conversational text format, it's not just numbers like traditional product analytics, so it can directly feed back into the AI.
The thing with LLMs, is that they are super powerful and general, this is the exact reason we want to use them that, they can answer any questions and execute a large range of tasks, however exactly because of that, their surface area to the real world usage is extremely large, the more they do the larger it is, resulting in countless different ways it can fall short. It is hopeless to try and predict all the ways your users will want to use your product, and try covering all the possibilities and edge cases beforehand.
How can a Data Flywheel help LLM apps?
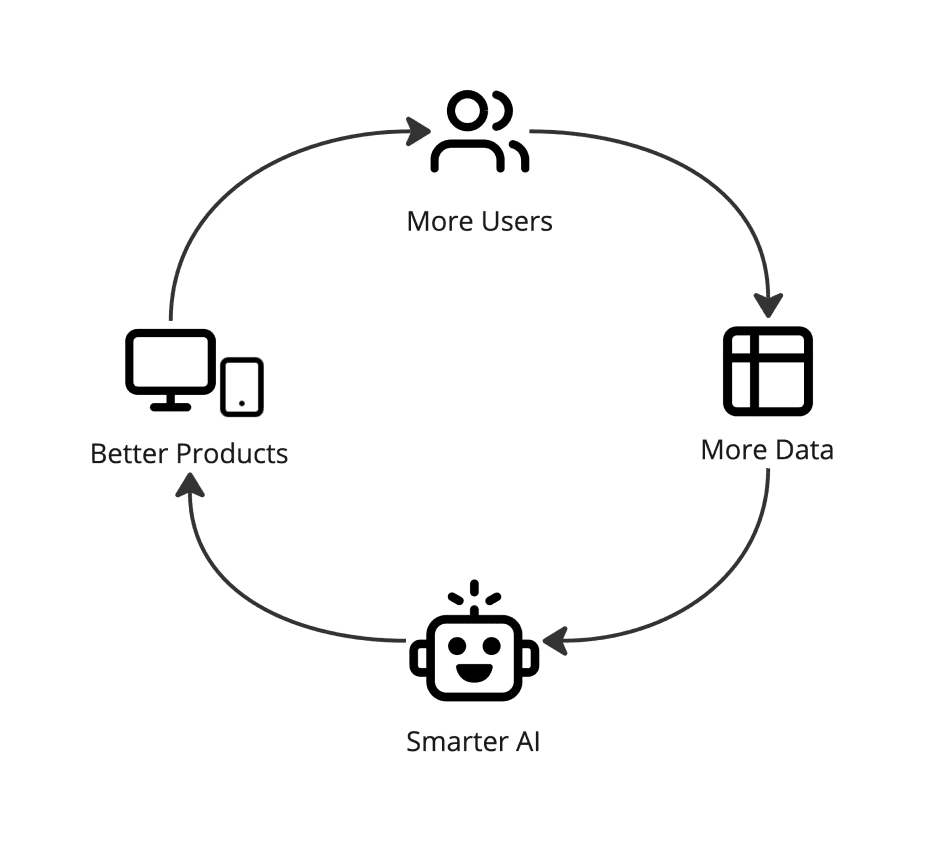
We call it a flywheel when an improvement brings business value and that loops back on further improvements. Since the number of possibilities of an LLM product is too vast - think of all possible ways a user can try using your product, or even compose a sentence - nothing is better at selecting what is more valuable and relevant like the real world, this makes it essential to monitor and get insights on where the product is suboptimal, or where it could bring even more value.
Then by using those insights for improving the product and making it more valuable, it will receive more usage and more users, it will gain more trust and cover more cases to be used in more places. By getting more usage, even more scenarios are revealed, new paths to bring even more value, and new shortcomings to overcome and make the product more robust, which will lead to even more usage an so on. That’s the flywheel.
The advantage with AI over traditional software improvements, is that this connection is even more direct, LLMs can be used also to evaluate, classify and synthesize data that will improve the product, so this flywheel is closed even tighter together.
There is, however, no shortcuts, if your solution has been finding new unexpected edge cases for months or years, it is not possible for someone else to just guess which ones they are and port them over elsewhere without going through the same discovery process. The improvement process you done on your product for your specific domain then creates an edge that sets you apart from others as soon as you start it.
LangWatch
LangWatch was built on top of this realization, to help you maximize your data flywheel. Our platform helps both on collection part, with monitoring, evaluations, insights, collecting user feedback and annotating with domain experts from your team, and on the improvement part, running experiments, validating and guaranteeing the improved quality of each iteration.
Interested how it can help your GenAI solution? Book a demo with us.
Boost your LLM's performance today
Get up and running with LangWatch in as little as 10 minutes.
Benefits
Features
Boost your LLM's performance today
Get up and running with LangWatch in as little as 10 minutes.
Benefits
Features
Boost your LLM's performance today
Get up and running with LangWatch in as little as 10 minutes.
Benefits
Features